Table Of Content
The advantages and disadvantages of these two approaches are the same as those discussed in Chapter 5. The between-subjects design is conceptually simpler, avoids order/carryover effects, and minimizes the time and effort of each participant. The within-subjects design is more efficient for the researcher and controls extraneous participant variables.
Sustainability is one more factor to consider in interior design - The Atlanta Journal Constitution
Sustainability is one more factor to consider in interior design.
Posted: Tue, 31 Oct 2023 07:00:00 GMT [source]
1: Introduction to Factorial Designs
This would mean that each participant would be tested in one and only one condition. In a within-subjects factorial design, all of the independent variables are manipulated within subjects. All participants could be tested both while using a cell phone and while not using a cell phone and both during the day and during the night. This would mean that each participant would need to be tested in all four conditions.
Factorial Design
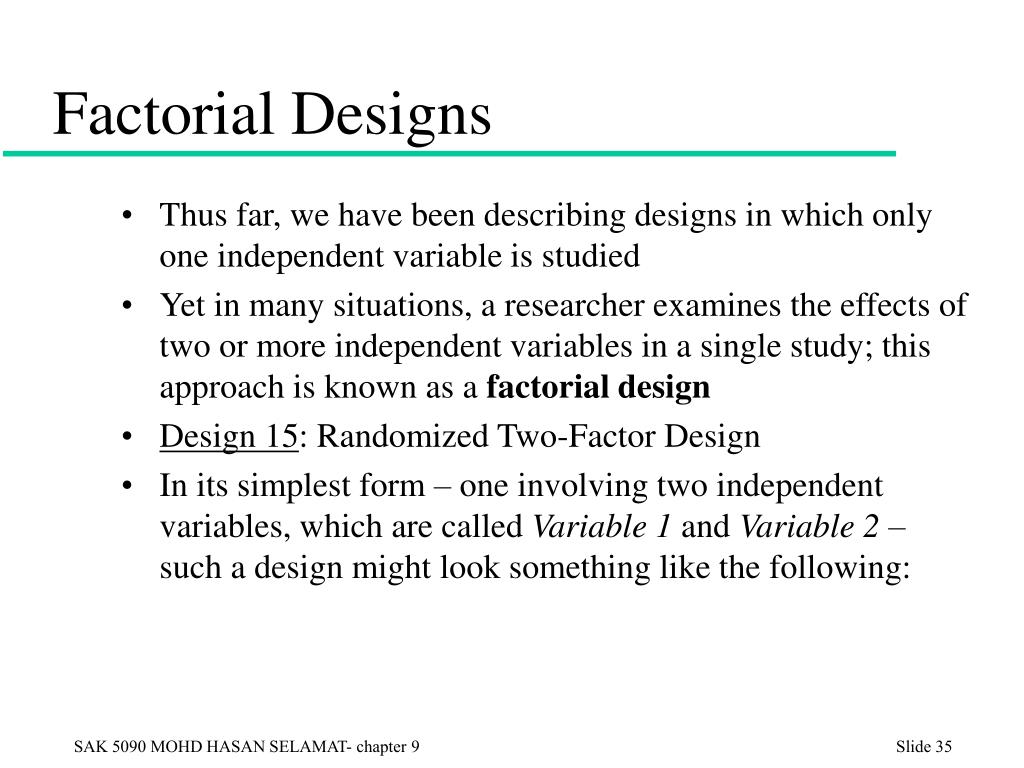
In such a design, the interaction between the variables is often the most important. This applies even to scenarios where a main effect and an interaction are present. A factorial design is a type of experiment that involves manipulating two or more variables.
A Catalog of Orthogonally Blocked Three-Level Second-Order Designs With Run Sizes ≤ 100
In any case, your mom has to consider both the fertilizer type and amount of water provided to the plants when determining the proper growing conditions. In the "Modify Design" menu, users can modify factors, replicate design, randomize design, renumber design, fold design, and add axial points. To change the factors, click the "Modify factors" radio button and then "Specify" to see the following options menu. Minitab 15 Statistical Software is a powerful statistics program capable of performing regressions, ANOVA, control charts, DOE, and much more. Minitab is especially useful for creating and analyzing the results for DOE studies.
We have usually no knowledge that any one factor will exert its effects independently of all others that can be varied, or that its effects are particularly simply related to variations in these other factors. The last four column vectors belong to the A × B interaction, as their entries depend on the values of both factors, and as all four columns are orthogonal to the columns for A and B. Belong to the A × B interaction; interaction is absent (additivity is present) if these expressions equal 0.[13][14] Additivity may be viewed as a kind of parallelism between factors, as illustrated in the Analysis section below. Researchers want to determine how the amount of sleep a person gets the night before an exam impacts performance on a math test the next day.
2.6. Main Effects and Interactions¶
Optimization of biodiesel production from castor oil using factorial design - ScienceDirect.com
Optimization of biodiesel production from castor oil using factorial design.
Posted: Wed, 29 Nov 2017 04:02:41 GMT [source]
If interactions are found, and inferential statistics must be used to unpackage such interactions, such simple effects tests would require examining the effects of ICs in only subgroups of the sample. In essence, if it is necessary to follow-up an interaction by identifying which particular subgroups differ from one another, some of the efficiency of the factorial design may be lost. However, it is important to note that interaction effects can be highly informative without simple effects tests (Baker et al., 2016; Box et al., 2005). The difference between red and green bars is small for level 1 of IV1, but large for level 2.
You can pick your two levels low and high close together or you can pick them far apart. As most of you know from regression the further apart your two points are the less variance there is in the estimate of the slope. The variance of the slope of a regression line is inversely related the distance between the extreme points. You can reduce this variance by choosing your high and low levels far apart.
Effects and Interaction Plots
Note that this is not a new level to our Distraction IV, but a whole new IV. In one condition, people will get $5 for every difference they find (so they could leave the study with lots of money if they find lots of differences). In the other condition, people will get no money, but they will still have find differences (comparison control condition). Imagine that the results show that people found an average of four differences when they were distracted, and an average of 10 differences when they were not distracted. The effect of distraction is a mean of six differences found in the picture.
This kind of design has a special property that makes it a factorial design. That is, the levels of each independent variable are each manipulated across the levels of the other indpendent variable. In other words, we manipulate whether switch #1 is up or down when switch #2 is up, and when switch numebr #2 is down. Another term for this property of factorial designs is “fully-crossed”. Other useful exploratory analysis tools for factorial experiments include main effects plots, interaction plots, Pareto plots, and a normal probability plot of the estimated effects. So, for example, a 4×3 factorial design would involve two independent variables with four levels for one IV and three levels for the other IV.
Besides the first row in the table, the main total effect value was 10 for factor A and 20 for factor B. However, since the value for B is larger, dosage has a larger effect on percentage of seizures than age. This is what was seen graphically, since the graph with dosage on the horizontal axis has a slope with larger magnitude than the graph with age on the horizontal axis. Experiments that include more than one independent variable in which each level of one independent variable is combined with each level of the others to produce all possible combinations. You might be wondering how this scenario on distractions and Spotting the Difference is related to factorial designs and ANOVAs since a t-test would be great at analyzing this data.
When we use a 2×2 factorial design, we often graph the means to gain a better understanding of the effects that the independent variables have on the dependent variable. Again, because neither independent variable in this example was manipulated, it is a non-experimental study rather than an experimental design. This is important because, as always, one must be cautious about inferring causality from non-experimental studies because of the threats of potential confounding variables. For example, an effect of participants’ moods on their willingness to have unprotected sex might be caused by any other variable that happens to be correlated with their moods. For instance, some interactions may be due to the overall burden due to subjects receiving large numbers of components. This might result in subadditive or negative interactions in which interventions produce less benefit, or even produce net decreases in benefit, when they co-occur with another intervention(s).
It is important to note that interpretation of complex higher order interactions may not be aided by simple effects testing. First, it is highly unlikely that such testing would have distinguished amongst the three leading combinations shown in Figure 1 (the differences in outcomes are too small). Second, such tests would have been grievously underpowered, and increasing the sample size to supply the needed power would have compromised the efficiency of the factorial design (Green et al., 2002). This, of course, has limitations, such as not permitting strong inference regarding the source(s) of the interaction. Alternatively, an investigator might modify an intervention when it co-occurs with a particular, second intervention component. For instance, assume that a design has three factors; two are medication factors (e.g., varenicline, on/off, in one factor and NRT product [nicotine patch vs. nicotine lozenge], in a second factor).
We've used Minitab to create the factorial design and added the data from the experiment into the Minitab worksheet. First, we will produce a normal probability plot of the effects for this data with all terms included in a full model. Having included all the terms back into a full model we have shown how to produce a normal plot. Remember that all of these effects are 1 degree of freedom contrasts of the original data, each one of these is a linear combination of the original observations, which are normally distributed with constant variance.
For example, instead of conducting one study on the effect of disgust on moral judgment and another on the effect of private body consciousness on moral judgment, Schnall and colleagues were able to conduct one study that addressed both questions. But including multiple independent variables also allows the researcher to answer questions about whether the effect of one independent variable depends on the level of another. This is referred to as an interaction between the independent variables. Schnall and her colleagues, for example, observed an interaction between disgust and private body consciousness because the effect of disgust depended on whether participants were high or low in private body consciousness. As we will see, interactions are often among the most interesting results in psychological research.
No comments:
Post a Comment